Big data: what is it and how’s it used?
April 4, 2023
9 min
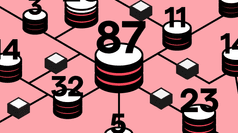
What is big data? In short, it is the immense amount of information that the internet generates, such that it exceeds the capabilities of the tools designed to analyse and store it. To better understand what it is and what it is used for, let us take a few examples and seek a more precise definition.
What is big data: meaning and definition
According to recent research by statista.com, in 60 seconds on the internet, 16 million messages and more than 230 million emails are sent, around 6 million Google searches take place, and more than 90 million dollars worth of cryptocurrencies are bought.
These actions bring with them such a large amount of data that they are called big data or megadata; however, there is no ‘minimum’ size for this definition. This threshold, if it existed, would change over time as volumes grow exponentially: for example, it is estimated that 120 zettabytes (1 billion terabytes) will be generated in 2023 alone, compared to 97 in 2022 and 180 in 2025.
The definition of big data, therefore, is ‘dynamic‘: it responds to the case where the information is so complex that new technologies must be created in order to store and process it in an acceptable time frame. The possibility is concrete, indeed it is already a reality, because the evolution of hardware and software does not follow the pace of data growth. Capturing and processing them has always been a challenge, so much so that as early as 1958, IBM coined the term ‘business intelligence’ to refer to the ability to understand the relationships between data in order to guide future decisions.
In 2001, Douglas Laney created the ‘3Vs’ model, listing the characteristics of big data:
- Volume – the amount of data that different sources produce, from social networks to the sensors of the Internet of Things, to purchases on crypto exchanges and marketplaces for NFTs;
- Variety – there are different types of online information, but it is possible to divide them into structured and unstructured. Sometimes the nuance of ‘semi-structured’ is also considered, which is a level with mixed qualities;
- Velocity – instant communication generates a very high flow of information per second, so we need tools that can capture and analyse it ‘in real time’.
This three-dimensional diagram over time has been supplemented with other parameters. First of all, we have the fourth V of Value: analysing the activities of online users represents an opportunity for profit, companies for example translate them into targeted marketing campaigns or forecasts on the future of the industry.
In this context, the degree of reliability contributes to the definition of big data: we find the fifth V in the Veracity of the information, which is fundamental for making useful and accurate estimates. In addition, we can recognise a certain Variability in data formats, essentially the variety in relation to time, and finally a Visualisation is needed to explain them, i.e. graphs and tables.
Where is big data collected?
We have no less than 7 Vs to express big data, but for some scholars, this does not exhaust its meaning. In practice, in fact, it is possible to highlight other characteristics, resulting from the processes of storage and analysis.
Fun Fact
The term big data was coined by John Mashey in 1998 and presented in a series of slides, called “Big Data … and the Next Wave of InfraStress.”
For instance, the comprehensiveness of the information is assessed, based on how many and which sources have been taken into account. According to another definition of big data, in fact, their complexity is such that it would be impossible to understand them if examined in smaller portions. In this regard, it is useful to elaborate on the difference between structured and unstructured data: in the former case, the properties and format of the information are predefined, whereas in the latter, a table with ‘fixed fields’ cannot be constructed.
In other words, the characteristics of structured data are already known: transactions, for instance, will always have a date, a time, a sender, a receiver and an exchanged value. Unstructured data, on the other hand, are contents that are impossible to catalogue systematically: images, video, audio and text, always different in shape and size. Examples of unstructured big data, therefore, are posts on Instagram or tweets and are estimated to account for 80% of the total.
Given these differences, there are two types of databases for storing and analysing big data:
- Data warehouses : useful for data that are already structured and have therefore passed the ETL process (Extract, Transform, Load), i.e. the extraction and transformation prior to loading into the database. Basically, the information is already ‘cleaned’ of redundancies and organised by relationship, thus ready for investigation.
- Data lakes: collect unstructured, raw and unfiltered data, which will only be sorted at the time of eventual analysis. This approach is less expensive in operational terms, but requires more space. Cloud storage technology is suitable for this purpose and, above all, is low cost.
Companies, however, prefer to combine the functionalities of the two types of databases into a single data lakehouse, suitable for both structured and unstructured data, for greater efficiency and reliability.
The meaning of big data, therefore, also passes through granularity, i.e. the level of detail of the information. In practice, represented in a table, this aspect measures how many ‘quality’ columns exist for each element entered in the rows. In this respect, these databases must be scalable and extensible: in a nutshell, respectively, we should be able to add new elements and fields to ‘fill in’.
What big data is for: examples and applications
Now that we have started to understand what big data is, let us also try to shed some light on its functionality. The purposes of megadata are essentially three and derive from different types of analysis conducted on them:
- Descriptive – studies the status quo or past phenomena, summarising and representing data in graphs and formulae, looking for relationships that link them;
- Predictive – statistical methods, in this case, are applied to big data to make predictions about the future. It does not merely describe reality, but looks for its possible causes in order to anticipate forthcoming events, which could repeat themselves according to the same mechanisms;
- Prescriptive – derives from predictive, because it is forward-looking and provides guidelines and optimal solutions to address specific problems.
These analyses are conducted by data scientists but can be assisted by artificial intelligence, which uses big data as ‘training samples’ for machine learning. In addition to describing and relating the available information, in fact, AI is able to predict next occurrences, based on laws discovered during analysis. In particular, structured data are the basis for ‘supervised’ tasks (such as linear regression), in which AI is guided by ‘labels’, whereas unstructured data are applied to unsupervised algorithms (such as clustering), because they do not need any guidelines to conduct searches.
Knowing the types of analysis and the role of machine learning, we can then understand how companies use big data and what it is used for. First of all, megadata is collected and analysed to create predictive models to anticipate user demands. In practice, products and services are designed based on the most successful online features, trying to meet customer demand. Netflix or Disney+, for example, evaluate opinions on the first season of a series, observe interactions on social media, so as to plan subsequent ones based on popular features.
Similarly, companies can exploit big data to improve the customer experience: essentially, to generate positive impressions in users during interaction with products or services, so as to retain them or acquire new ones. Examples of big data, useful in this case, are feedback collected through simple forms, reviews in app stores, but also comments on social posts. Finally, from the analysis of data, it is possible to detect fraud attempts, which might follow recurring patterns, so as to improve the security of platforms such as exchanges.
Outside the world of the Internet, we find other examples to explain what big data is for.
- Predictive maintenance: sensors that monitor the operation of cars produce unstructured data, accompanied by structured information such as year of registration, model or fuel type. Both examples of big data are useful to predict possible breakdowns, so that maintenance can be planned in advance.
- Management efficiency: in the industrial sector, megadata analysis is useful to improve processes in the production of consumer goods. For example, it is possible to monitor ‘return’ rates and general market demand in order to optimise future production.
These applications help us understand what big data is, but they also have important privacy implications. Current regulations oblige companies to warn customers when they would like to collect their data. Moreover, it should be possible to refuse: for example, every web page manages consents through cookie options, the files that record users’ preferences.
In the past, the misuse of big data has led to scandals such as Cambridge Analytica, which allegedly exploited information linked to many Facebook profiles to benefit Trump during the 2016 election. However, the application of blockchain technology to the internet, represented by Web3, should solve the problems of data ownership, giving new meaning and definition to big data.